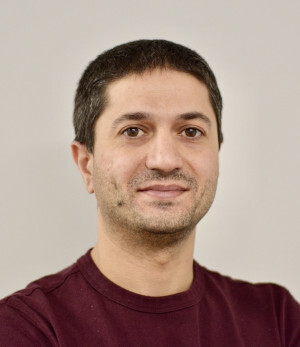
I am a postdoctoral associate in the van Rees lab at the Department of Mechanical Engineering, developing algorithms for fluid mechanics and FSI problems. Moreover, I leverage deep learning to build a digital twin framework to understand dynamical systems in fluid flows. Before joining MIT, I was awarded the Fields CQAM postdoctoral fellowship at the Fields Institute at the University of Toronto, where I worked on reduced-order modeling for fluid dynamics in a Lagrangian system. Through my research career, I have honed my expertise in using physics-based machine learning algorithms to develop surrogate models for large-scale Partial Differential Equations (PDEs) and PDE-constrained optimizations. I am particularly interested in the intersection of chaos theory, optimization, and machine learning, where I study the impact of chaos on universal laws in fluid dynamics. For further information, you can visit my website: https://hkarbasian.github.io